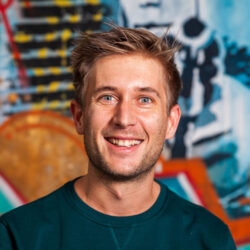
Data Centric AI is about iterating on data instead of models to improve machine learning predictions.
Why is this trend relevant now? Is this yet another hype in data science? Or has something really changed?
Traditionally, data scientist tend to treat their datasets as static and focus on improving their machine learning model.
And that’s not so strange:
Because that’s what they do in academia
What they learn in courses
It’s what most online competitions focus on
Where most tools are being built for
This narrow focus has a name: "model-itis"
But something is changing. Machine learning models are being commoditized. Look at HuggingFace, they’re crushing it with models for text, vision and audio. Your data scientists can’t compete with that. The competitive advantage of data scientists lies in everything that surrounds the model.
What should your data scientists do instead? Focus on the things that don’t scale outside your organization:
Define great metrics
Develop high quality datasets
️ Discover systematic errors in your models
Ship models to your users
…and more
Your data is unique to your use case. Improving your data can be easier and have more impact than focusing on the model.
Improve your label quality is something you can do straight away. The course Machine Learning Engineering showed that clean datasets need way less training data than noisy datasets. Label quality makes a huge difference.
Not all of this is new. The ecosystem around SpaCy has had a focus on iterating on code and data for years. But the wider appreciation of this focus is new.
What’s my favorite benefit of Data Centric AI? It makes it easier to collaborate! Model Centric data scientists tend to get stuck in their ivory tower. Data Centric data scientist talk to domain experts and ask how the data got generated… and that’s where the real learning is.
Now if you’re a data scientist and think: "But I actually like working on the models". Then don’t despair! Because we’re in dire need for better tools that make it easier to iterate on the data. And models play a role there too!