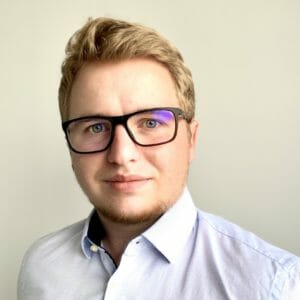
Recently, I was invited as the main guest on the DataTalk podcast, a series of podcasts about important data and analytics topics with data science leaders from around the world. In this episode, I talked with Mike Delgado, Experian’s director of social media, about Data Science Challenges for Non-Tech Companies and how to overcome them.
Furthermore, you can find the episode on the Experian website and in iTunes. You can also subscribe to the DataTalk podcast in iTunes.
In my daily work, I come across many organizations that aspire to overcome their data science challenges. Besides this topic, Mike and I talked about more things:
- Tips for aspiring data scientists to make a successful transition from academia to industry;
- My daily work as a Chief Science Officer and Director of Training and Development;
- Competencies and traits of data scientists, and why traditional companies struggle to find them;
- How do you make your organization attractive for data scientists;
- And more.
Each topic could be a blog post, but we can delve a bit in to the main one: data science challenges and how to solve them!
Common Data Science Challenges of Businesses
When I look at the companies around us, I mainly see them struggling with:
- Hiring the right profiles;
- Centralizing data and automating its movement;
- Finding the advanced analytics use cases that add real value to the company.
There is no particular order here, because they are all related: having two of the three is not enough!
If you don’t have use cases or data: well, it all ends there.
If you have the best use case and the data available, but no data science capacity to actually deliver something working to your engineering team: you won’t be making a penny for your company.
This means that you could still have data scientists, but as long as they’re not able to contribute to building data products, you’ll have a hard time getting value out of them.
Solving the Data Science Challenges
To solve the challenges, I talked about the business/analytics translator role. This relatively new role bridges the gap between the business and the teams, by translating business requirements in data products.
Then I talked about the importance of data engineering as a fundamental building block for all data driven organisations and how learning journeys (more on that in a future blog post) can help you grow your talented people from within the organisation.
And to close it, I talked about how being open at the core, with contributions to the open source ecosystem on one side and publishing of research papers on the other, helps attract talent from the outside. If everything else is levelled out, open companies will always be more attractive than their more closed-up peers.
End of the spoiler! If you want to know more, head over to the Experian website to hear it all.
Are You Ready to Solve Data Science Challenges?
Join us for the Data Science for Product Owners course. This training teaches you all about creating AI solutions that drive value and allows you to bridge the gap between data teams and business users.
If you want more rambling throughout the month, follow me on Twitter: I’m gglanzani there. But the real fun is on LinkedIn where I always search the boundary of the professional social network!