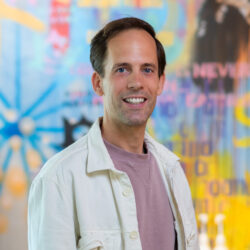
The data landscape is constantly evolving, making it challenging to stay updated with emerging trends. That’s why we’ve decided to launch a blog that focuses on the data trends we expect to see in 2025.
1. The Future of Data products: Empowering Businesses with Quality and Governance
As GenAI is in transition from a hype to a mature product, the realization of the value of data quality has re-emerged. Data governance is rapidly rising on the priority lists of large companies that want to work with AI in a data-driven manner. Poor data quality automatically results in poor decisions. That applies not only to GenAI but to all data products.
In many companies, data is spread across different storage locations and platforms, thus, ensuring effective connections and governance is crucial. The key to building successful data products is understanding the needs and challenges of the business that the data products aim to address. By 2025, we will place responsibility for the data in the hands of those who know it best: the business teams. If we want users to fully leverage the potential of data, they must gain insights into the semantics, quality, and lineage of all data. As a result, the following data resources will become more and more important:
- Data contracts
- Data catalogs
- Data quality and observability tools
- Semantic layers
One of the most important questions will therefore be: How can we make data optimally accessible to non-technical users within organizations?
2. Empowering Business Users: The Next Frontier in Data Democratization.
Tools like dbt accelerated data democratization by allowing engineers to “shift left” business logic and create a hub-spoke model for data. This results in increased efficiency and trust in data quality (well, or at least uniformity of business logic across various dashboards).
Typically, an analyst feeds this data in a dashboard for business users. Irrespective of how much drilling a dashboard allows, business users will turn to data specialists with data questions. Data teams are not known for their empty backlogs, implying a bottleneck for ad-hoc business questions.
The next step in data democratization is to allow business users to directly interact with data in the datalake or datawarehouse in natural language. No more bottlenecks nor a need to learn SQL. Tools to watch in this space are Databricks AI/BI Genie (Databricks AI/BI Genie), Snowflake Cortex Analyst (Snowflake Cortex) or domain-specific tools such as (Akkio ) for advertising agencies.
3. Unlocking Data Access: How Data Cataloging is Transforming Organizations in 2025.
Data cataloging is a trend that helps further democratize data across organizations in 2025. A data catalog is like a library management system in which data sets and data products are books and employees are library patrons. A library management system helps library patrons find and check out books. A data catalog helps employees find and use data (products), and thus is an important next step in data democratization.
Data cataloging helps users intuitively discover available data (products). Metadata such as titles, definitions, glossaries, tags and classifications helps to better understand the data (product). Lineage (i.e. the flow of your data) helps employees to better understand up- and downstream dependencies.
In addition, data catalogs make data access management more intuitive.
A data catalog centralizes knowledge about data (products), empowering business teams to take responsibility for the data products that they provide to the rest of the organization. Instead of IT being responsible for all data (products) without having influence on the data generating process, data owners and data stewards in business domains can now be made responsible for data definitions and the quality of their data (products).
Some vendors to watch in this space: (Atlan), (Collibra ), Databricks Unity Catalog (Unity Catalog), Informatica Data Catalog (Informatica Data Catalog) and Talend (Talend Data Catalogue). And (data.world), a company that we are particularly interested in because of their knowledge graph architecture.
4. The Rise of Agentic AI
By the end of 2025, agentic AI will revolutionize how companies automate complex tasks and make decisions. Unlike traditional AI systems, agentic AI operates autonomously, tackling processes like HR management, customer service, and even financial transactions. These “digital workers” will fill roles historically reserved for humans, providing capabilities like resolving support tickets, assisting in human resources, and automating complex workflows. Industry leaders like Microsoft, Google, and Salesforce are leading this shift, forcing SaaS providers to embrace agent-based models to reduce operational costs and improve efficiency.
Despite the promise, obstacles remain. AI agents remain prone to error, and significant missteps could result in public and regulatory scrutiny. The first agents to emerge are expected to perform small, structured internal tasks with some degree of fault-tolerance, such as helping to change passwords on IT systems or book vacation time on HR platforms. Businesses must prioritize robust security, monitoring, and ethical standards to ensure these systems are deployed responsibly.
Organizations that embrace agentic AI early are set to gain a competitive advantage. By boosting productivity and fostering innovation, human-AI collaboration will reshape workplaces, making operations more efficient, scalable, and adaptable. To get started, companies should begin with low-risk, structured tasks where AI can provide immediate value while minimizing potential errors. Implementing strong oversight mechanisms, such as human-in-the-loop validation and continuous monitoring, will be crucial for risk mitigation. Additionally, investing in employee training and establishing clear ethical guidelines will ensure a smoother transition. By taking a measured, strategic approach, businesses can build a solid foundation for AI-driven transformation while maintaining trust and compliance.
5. Evolving roles and competencies
The ‘data scientist’ role was once considered the sexiest job of the 21st century. However, this position, which traditionally encompassed a wide range of skills, roles, and tasks – is now evolving. We observe that the skills, responsibilities, and tasks of data scientists and machine learning engineers are increasingly overlapping.
Today, a data scientist must develop reliable, scalable, and maintainable machine learning pipelines using best practices from software development and be able to effectively communicate results to business stakeholders. Furthermore, with the rise of LLMs as decision making tools, you will need a data scientist in order to be able to guarantee reliable, valid, and robust results.
6. GenAI in 2025: Security, Observability, and the further rise of LLMOps
The initial hype surrounding AI is evolving into a focus on practical applications that deliver measurable value. Businesses now understand what AI can actually do, moving beyond early excitement. The real challenge in 2025 is using AI effectively and responsibly, which is where LLMOps (LLM Operations) comes in. LLMOps can be seen as a way of streamlining the development, management, deployment, and monitoring of LLM applications. It’s the toolkit for reliable, safe, and value-generating AI. Here, security will remain the top priority. Robust safety measures are essential for deploying generative AI effectively. Strong guardrail mechanisms should be in place at all costs, actively validating both inputs and outputs of the system.
Likewise, understanding AI performance is essential. "Observability" gives us a control panel view, using real-time dashboards, logs, and data tracking to swiftly resolve issues. Data-driven evaluation, using specific metrics for accuracy, factuality, and bias, replaces intuition. Human experts annotate data, address edge cases, and ensure high-quality evaluation, providing the insights needed to further improve the system. Companies that understand how to evaluate their system efficiently and effectively will see the largest value gains. Moreover, successful deployment of generative AI will require both a solid understanding of Generative AI as well as best practices from software engineering. For many companies, scaling generative AI is about orchestrating calls to pre-trained models and other APIs. Engineering efforts are now centered around optimizing costs and building robust, scalable applications that balance speed, accuracy, and efficiency. A strong foundation of platforms and tools is critical for realizing the full potential of Gen AI in 2025. This foundation not only enables responsible and impactful use but also drives business value: accelerating time-to-market for new products, shortening iteration cycles to enable faster product improvements, while significantly mitigating the risk of negative consequences. Companies with robust AI lifecycle management will be positioned to lead in innovation and achieve a significant competitive advantage.