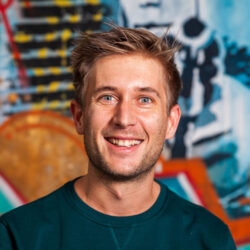
Beyond the numbers: how a solid business case drives successful analytics
Guidelines on writing your business case
Successful analytics projects have a clear financial driver. The reason for initiating such projects often is to increase efficiency, either by reducing cost or increasing revenue. However, many companies fail at running successful analytics projects. A key factor is that focus on delivering true business value is lacking, and that expected benefits of the use cases are not tracked well during and after execution.
The Xebia value chain of data science (find a description of the value chain here: Defining Analytics Use-Cases) describes how to transform data to business value. This already makes sure you understand how business value will be realised, before starting your project. However, I’d like to take it a step further by always adding a business case to this. A business case makes sure that you’ll:
- Create a deeper understanding how effort and benefits should be balanced to stay profitable
- Have a clear idea about financial expectations in your communication to stakeholders
- Allows you to track the value during and after development to keep evaluating if it’s worth continuing the investment
Factors that drive business value
When talking about data and analytics projects, I’d like to use the term ‘use case’. A use case is the end-to-end delivery of a valuable product to end users, such as a dashboard or analytical model. In this case, end-to-end means all steps between ingesting a data source, to building data transformations, to developing the product used by end users. Those products at the end of the chain should
There are many factors that play a role in the end results of your data and analytics use cases. We divide them into three categories:
- Business or process drivers; the degree to which the solution solves the business problem, for example the reduces time spent on manual tasks. This depends on the extent to which the solution automates a process and/or a decision.
- Solution adoption drivers; for example, the number of active users. This says something about the degree to which users are effectively using the solution – and depends on training, communication, implementation plan, and solution-user fit.
- Model drivers; such as accuracy, precision and recall. This describes the performance of your analytical model.
Aside from use cases that generate direct business value, there are also factors that contribute to the development of the general data and analytics capability. A more mature capability will result in decreased time-to-market of use cases and increased adoption rates. We call those factors ‘enablers’, they contribute value creation indirectly.
Examples of ‘enablers’ are the development of a data platform, the set-up of a data academy, or the development of a data management strategy. These initiatives don’t deliver value on their own, they deliver value through use cases. A data strategy roadmap should be focussed on both the delivery of use cases, as well as developing the strategy enablers.
An approach to writing a business case
How do you start writing a business case? The first step is to choose how to approach the business case. There are roughly two ways to do this; top-down or bottom-up. Which one to choose depends on the nature of your business case and phase of the project. Top-down is based on general ideas and assumptions, and bottom-up based on specific information.
Top-down approach
A top-down approach is about the big picture, many assumptions will be captured by a couple of variables. For example, the basis for your business case could be the market as a whole, focusing on the trends that are relevant to your company. The outcome gives an idea of potential, often more optimistic compared to the bottom-up approach. This approach is suitable when the project is at it’s very early start, in the exploratory phase. It provides an insight into the possibilities.
Once the solution idea has become more concrete, you should go a bit further and take into account the three categories mentioned in the previous section. By taking this route, you’ll go into a more bottom-up approach.
Bottom-up approach
A bottom-up approach is much more specific, and focusses on a company’s characteristics and processes. This approach requires a much higher level of detail and stakeholder involvement, it is therefore more time consuming. It will deliver a more realistic estimation of cost and benefit compared to the top-down approach, and it gives the opportunity to understand how individual factors contribute to the overall goal.
The image below shows a high-level example of how the three drivers, as well as strategy enablers, play a role in defining a business case for your data and analytics project.
The process of building and validating the business case
The development of a data and analytics project can be divided into three phases; ideation, experimentation and industrialization (see AI Use case generation for non techies). Each of these phases requires a different focus when it comes to the business case.
Ideation phase
In many organisations a positive business case is a prerequisite to start your data and analytics project. Take the bottom-up approach and involve relevant stakeholders in the process of building the business case. Note that the top-down approach is suitable for the phase before ideation, when there are no concrete solution ideas yet. Make sure that your assumptions are recognized, so that your business case is support by decision-makers.
To make your storyline work, include the following:
- How does your data and analytics project add value
- Explain the baseline situation you’re comparing to, e.g. a manual process
- Quantification of business value over time, and timelines on the break-even point
- What assumptions did you make
- You might take uncertainty about the assumptions into account, and present several scenario’s (progressive, neutral and conservative) to give an idea of bandwidth
- How will you validate those assumptions, and track them in practise
The final step in this phase is to determine benefits and cost to ultimately answer the question: “Is it worth the investment?”. Keep in mind that a business case is an estimation, highly dependent on your assumptions. Use the business case to determine to go for the idea or not.
Experimentation phase
This phase is all about validating the assumptions and adjusting the business case if necessary. During this phase you’ll experiment with the solution and pilot it with a first group of users. This way you’ll get a realistic understanding of what’s possible or not. Make sure not only to focus on the model accuracy, especially a pilot will give lots of insight into the adoption and business drivers.
Compare the estimated assumptions from the ideation phase, with the validated assumptions from this phase. See if these new insights fit with the progressive, neutral or conservative scenario. Make sure to use the validated assumptions to update the business case.
Share these insights during a progress update with your stakeholders. Explain how the validated assumptions affect the business case. Use this information to determine to industrialize the solution or not.
Industrialization phase
After productionizing your data and analytics project, keep monitoring key drivers. Especially if the business case will reach break-even after some time, it’s important to keep track of assumptions of all three driver categories. This will give you the possibility to act in case of decreasing (or increasing!) results.
Conclusion
Crafting a business case for data and analytics projects is essential for securing buy-in and ensuring long-term success by creating insight in the expected and actual value. It also gives a fact-based approach to starting and stopping investments. Start thinking about what true business value means to your organisation, and focus on tracking it before, during and after developing a data and analytics solution.