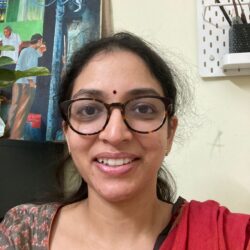
With the huge popularity of AI Tools like ChatGPT and Bard, many tech companies have started building AI tool customizations like Chatbots. Since the insurance sector across the world is growing steadily after the pandemic, these tools have started entering the InsurTech market too. However, before implementing and using AI extensions, it is good to be aware that these have limitations. One needs to understand and address these limitations to broaden the tool’s usage. When you take appropriate care right from the design stage, you can add new AI functionalities to your products and make them reliable too.
Here are 5 tips to start with.
- Define and implement Guardrails. When we add an AI chatbot to an insurance platform, we need to make sure that only relevant questions are asked. There are two ways to do this. Either we can limit the questions by replacing text boxes with pre-defined questions or train the chatbot to point out and skip irrelevant questions. Though the first option is easier to implement than the second one, when text input options are many (diverse intents and categories), the latter is preferable. Either way, since these chatbots are not generic like Siri, Alexa, or ChatGPT, it is important to define and enforce boundaries.
- Use it not just to automate customer service, but also further security and privacy. Since LLMs used in the insurance sector have access to sensitive information like customer contact, medical information, asset details, etc. it is important to gauge security loopholes that may pop up. A good practice is to evaluate threats and use LLMs to answer them. It is important to note that security can’t be relegated to the end but should be thought of during the initial ideation stage.
- Strengthen data structures as they form the basis of LLMs. Since LLMs learn from the data supplied, form connections, and provide analysis, it is extremely important that data is verified and structured appropriately. Additionally, there should be tight security measures like timely audits, and role-based access control. In fact, if not for the imperative security measures, implementing LLMs is fairly easy. However, before LLMs are added to applications, it is important to ensure data privacy with appropriate data security measures.
- Provide thorough training to the models for efficient and ethical implementations. As with humans AI tools are not without biases. Makers should be conscious of these biases and take appropriate measures to prevent transferring human biases to LLMs. For example, insurance premiums can be calculated based on actual risk, but not perceived risk falsely calculated according to insurers’ surnames, race, gender, etc. Companies building smart software products should employ people from diverse backgrounds to build and test LLMs. They should include bias tests in regular product tests.
- Use it along with other technologies like LDMs (Large Data Models) and NLP (Natural Language Processing) to make more advanced operations feasible. Technology upgrades like using LLMs in Chatbots or customer care automation usually affect various components in the product or platform. Sometimes, functions of LLM implementations may intersect various departments of the business. It is thus beneficial to think about combinatorial technology implementations. Businesses need to think, along with leveraging LLMs, what other technologies can be explored, what upgrades can be implemented together, and what functionalities can be grouped and added to their product and service offerings.
LLM implementations may seem deceptively easy and simple. However, they affect critical aspects like security, data privacy, business strategy, operations, etc. A small mistake, even though unintentional, may have serious ramifications and turn out to be too costly for a company. When choosing a development partner for LLM implementations it is important to consider their experience in various domains and technologies, not just in AI tool development.
coMakeIT as a part of its parent organization, Xebia, builds reliable and scalable software products for businesses in diverse domains and across regions. With about two decades of experience, coMakeIT offers its customers not just product and platform development knowledge, but also a holistic technology vision from its expertise in deep analysis, strategic planning, and product thinking. If you’re looking for a secure and reliable implementation of novel technologies like LLMs and AI for any domain, please talk to us.