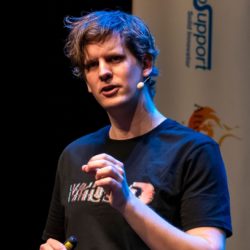
With the huge popularity of ChatGPT, many software businesses are trying to incorporate AI abilities into their products or service offerings. The Insurance sector too is not any different. Compared with other domains like banking and education, the insurance domain was not very fast in digitalizing its data and workflows. This is not because it was not growing, but due to other constraints such as dispersed and multiple forms of data, paper-based workflows, privacy issues and regulations, etc. However, with the latest advancements in large language models (LLMs), Large Data Models (LDMs), and Natural Language Processing (NLP) various products are being built that answer the constraints and digitalize the sector.
These products are not merely digitalising operations and workflows, they also help insurance companies expand their services, increase profits, reduce costs, and work more efficiently. Moreover, they are evolving fast to address the changing needs of businesses and consumers. Since LLMs and other AI and ML tools are self-learning systems they help in bringing personalised products, minimising fraud, checking regulatory compliances, etc.
Here are some of the use-cases of LLMs in InsurTech.
- Chatbots powered with AI can be employed in logging customer service complaints, providing objective information about various services and the status of complaints. They are faster and more efficient. They help in reducing the load on customer care executives to help them concentrate on more important tasks.
- LLMs when fed with accurate customer data can provide a predictive analysis of individual customers or customer segments. They calculate risks better and suggest a more appropriate premium customized for each individual or group. The same customizations can be extended for processing renewals and claims.
- AI systems can be used to analyse Geo-locations, videos, and pictures. When this data is connected to LLM applications, they can understand scenarios such as the validity of claims and claim events, pre-evaluation for various risks, etc.
- LLM applications when embedded in various IoT devices can gather real-time data and adjust profiles based on it. For example, if the data collected indicates that a client is healthy and fitness-conscious, insurance firms may offer benefits and incentives like reduced premiums.
- Blockchain technology can be used for processing smart contracts automatically. Such applications are critical when unexpected events like natural calamities and huge accidents occur. LLMs and LDMs can be used in the process to communicate relevant details and automatically store and change relevant data accurately.
- LLM powered applications act as the face of various AI tools. Since they’re conversational, customers are likely to interact with them regularly. When AI tools are used to analyse the behaviours of clients, LLM tools like chatbots can be used to nudge customers to adopt better lifestyles, behaviours. This is both useful for insurers as well as insurance companies as they minimize claims.
- New ways of grouping customers arise, especially with groups formed by dispersed customers with similar needs and usage patterns. Companies can come up with new strategies for customer engagement by using AI tools and the analytics they provide.
- Chatbots can make regulations understandable and hence easy to comply with. Do you know any customer who remembers the terms and conditions that they agree to before buying a policy? Chatbots can be used to explain the important parts that are tricky or usually ignored. For insurance policymakers and providers, the same chatbots when trained on compliance laws can help them check regulatory compliance accurately.
- LLM tools can improve the transparency of contracts. Many times, claims are rejected because customers forget policy details. LLMs are good at summarizing complex texts like insurance contracts. They can explain various policies and provide a comparative analysis to customers as per their knowledge level and personas.
- LLMs and NLP applications are growing more advanced. Very soon, Chatbots will be able to answer customers not only accurately but also in their preferred language.
- LLMs can be used to streamline underwriting processes. They can extract relevant information from documents, enabling underwriters to assess critical factors such as risk faster and with greater precision. They can be used to check for conflicting language, typos, and grammatical errors in important documents. Such applications eliminate human errors and can reduce the anxiety of those responsible, especially because in the insurance domain, such mistakes can have serious consequences.
- LLMs can be used to automate text-heavy processes. Since language models have been proven to be quite good at making summaries of data in multiple forms of content like text docs, videos, and images, they are quite helpful in simplifying and clarifying various documents used and processes followed by insurance companies.
A good point to note is that all the above applications use a combination of technologies and not simple LLM implementations. A sector as complex as insurance can’t have too simplistic technology implementations. This is true for AI, LLM, NLP, RLHF, Retrieval Augmented Generation (RAG) or any other technologies. Machine learning, artificial intelligence, and NLP form the foundation of LLM implementations. It is hence important to choose a product engineering expert with deep knowledge of several technologies than someone who narrowly focuses on LLMs or domain specific GPT implementations.
Another important issue is that LLMs are not without negatives. Hallucinating Chatbots often make up facts or give irrelevant information. Generic applications like basic ChatGPT that are not meant to be used in serious domains can afford to make such mistakes, but a GPT implementation for a serious and complex domain like Insurance must not give any erroneous outputs. Wait for our next blog for some practical tips to prevent such errors. Meanwhile, if you are looking for an LLM implementation for any domain, or if you would like to know more about how LLMs can be incorporated into software products, please talk to us.