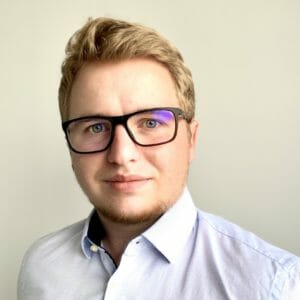
Digital technology can solve many issues for a variety of businesses and industries. There are many challenges that simply can’t be solved through real-world, physical means alone – we need digital solutions to give us the computational speed, insights and capabilities.
The Taguchi Methods are one such issue – but there also a great example of issues modern digital solutions can finally solve. Here, we – Łukasz Panusz and Maciej Mazur – want to talk about Taguchi Methods in particular, because we’ve been developing a solution for manufacturing that can finally resolve this challenge.
What Are The Taguchi Methods?
The Taguchi Methods are a series of statistical methods of improvement, as designed by the eponymous Genichi Taguchi in the 1950s. An engineer and statistician, his methods were developed to help improve production from a statistical point of view. While they have been used in countless sectors, they were primarily designed with manufacturing in mind.
A key part of Taguchi’s approach was the inclusion of loss functions – factors or values that can cause a detrimental effect (or ‘loss’) into the final product. Reducing one such loss function, without adding to another elsewhere, is part of the great statistical balance behind the Taguchi methods.
Manufacturing Problems In The 21st Century
Combined with the above factors, we can see the many problems industries have to solve. The most expensive production process creates loss in the form of revenue, while using cheaper materials can result in an inferior product that consequently sells poorly, resulting in further loss.
When these parameters are limited to a handful, human comprehension has long been able to suffice. For example, consider this chart, where we are comparing three different parameters:
As you can see, it’s easy to see the most optimal solution for the final product. However, what happens when we add a 4th or 5th factor? What about 100? There is no easy way to show 100 factors for human comprehension.
Additionally, with increased demand and necessity, companies are looking to produce their goods faster than ever. As such, while we have introduced conveyor belts, servos and more advanced equipment, the actual analytical and responsive side has lagged. Taguchi’s methods highlighted a fundamental issue – one that has only scaled with the industry – but the technology has only just caught up to meet these needs.
Digital Solutions
Fortunately, a computer can think many times faster than a human. Thanks to recent innovations, particularly in the Cloud, companies now have access to fast, responsive solutions that can be left to run almost entirely independently.
Here, we want to break down a few of the key needs that recent developments have helped to resolve.
Analytics
Before we can improve something, we must first know what is – and is not – working.
Companies have relied on data since the beginning of manufacturing. At first, it was enough to know how much could be produced in such a timeframe, and for what cost. But now, in a world with numerous suppliers, parts, production processes and elements, the need for greater analytical insight is paramount.
This requires two things, the first of which is a means to actually acquire the data. While companies can do this manually, this is too slow. The time taken will mean that any identified improvements won’t happen for a while – in the meantime, the current, inefficient process is still being used. This is where the Internet of Things (IoT) is proving most useful – enabling companies to get information on the fly, sorted and formatted automatically.
We’ve already done such work a for a few companies, including image recognition applications for the metal industry that is able to photograph products and give human workers exact information on particular elements not up to standard.
Secondly, this is then supported by powerful analytical tools. The requirements here are simple:
- They must be fast – if we’re getting data in at lightning speed, we need to find insights, correlations or other factors (such as Taguchi methods) just as quickly.
- Likewise, they need to be able to handle all the information at once – this is where Big Data technology comes in handy.
- The results need to either be something a human can understand – such as visual feedback, data correlations, or direct, automatic actions, which we’ll discuss shortly.
All of this, of course, is an endless cycle. As companies optimise one parameter, others may change, so advanced solutions are needed to balance all of these factors in a constant pursuit of perfection. Ultimately, we are able to find this perfect balance much faster and more effectively with machine assistance than on our own.
Digital Twins
We’ve spoken about Digital Twins before – especially as part of Industry 4.0 – so we won’t go overboard on details here.
Digital Twins – virtual replicas of real-world processes or machinery – enable companies to assess, analyse and experiment without interfering with actual production.
However, as we mentioned earlier, the modern industry has some growing needs. Digital Twins work on a smaller, singular level – such as one production nest, line or plant. But what about those with numerous plants working simultaneously? We need a solution that fits a global scale.
Mesh Twin Learning
The answer then, is to combine Digital Twins and advanced Machine Learning models through a mesh that connects different factories together. This mesh itself is powered by the Cloud, but we’ve created a solution that enables individual models to operate independently.
Here’s an example: we are making a product in different factories. The product is the same, so we want to know the best way to produce it. Each factory or production line has digital twins, taking local data and analysing it for improved insights. When a more optimal solution is found, the factory sends this new model to the Cloud, where it is distributed amongst all other factories, spreading the benefits.
Furthermore, we can use some experimental design to offer continued improvement beyond the industry standard…
Experimenting with Multiple Plants
Another benefit of this system is that, when an organisation has numerous plants creating the same product and process, it provides an ideal control group for experimentation. This is also something Genichi Taguchi thought of – we know it as Taguchi experimental design.
Let’s say your business has three factories and you want to test for potential improvements. If you have one factory tweak one parameter – let’s say temperature – while another tests something else – such as the amount of material – we still have a third factory to use as a control.
Furthermore, as we’ve already explained, any benefits can be then applied to the wider mesh. With this constant process, we can check and test all of the parameters individually, allowing us a wealth of statistical information. Taguchi would be proud.
What can we solve?
So, this is all sounds good in theory, but what principles can we actually solve here? Well, there are a whole variety of issues that can be addressed:
- Cost – can a cheaper solution be used without cutting quality or other factors that impact the final product?
- Environmental conditions – will a change in conditions enable a better or worse product?
- Material consumption – will changing certain materials, or the ratio of ingredients, improve the final goods in any way?
- Time – is there a shorter approach, with fewer steps or less repetitive processes? If it doesn’t impact the final outcome, then a shorter production time arguably means more goods can be produced.
- Volume – can we produce more at once?
- Cycle times – can we spin up more cycles, or will this impact other areas. For example, if production is faster and ran more frequently, would the over usage of machinery cause temperature or environmental conditions to change?
- Quality control – if there is a difference in quality, we can use the historical data to see which unique parameters caused it. Then, we can act to remove those issues and restore consistent high quality.
As you can see, there are plenty of issues to consider – and these are just a few. If you think how many components go into making a vehicle, and the amount of work needed at each stage, the amount of parameters to actually consider is staggering. A digital solution like Mesh Twin Learning is the only way to assess all of these plants and materials, as well as deliver actionable insights in a beneficial time frame.
Summary
The manufacturing industry is always evolving and adapting, so companies are always looking for adaptable solutions that scale with their needs. While Digital Twins and analytics work at a singular level, Mesh Twin Learning can help provide not just analytics, but also automatic adaptability, at the larger organisation level.
Business Perspective
With Mesh Twin Learning, manufacturing businesses can set-up systems that continually look to improve operations across countless factors, from cost to efficiency and consumption, all of which provide numerous unique benefits. However, once a benefit has been achieved, it can spread across the company as the new baseline, enabling the Mesh Twin Learning system to look for the next advantage.
Sources
- Taguchi Methods
- Taguchi Methods and How They Apply to Manufacturing
- Using Taguchi methods to improve the production process quality: A case study