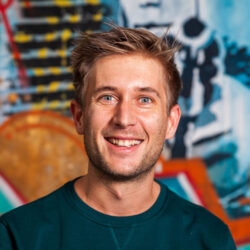
In the last few years, the discipline of Machine Learning Operations (MLOps) has been received a lot of traction to get more Machine Learning (ML) solutions into productions, reduce iteration cycles, and reduce costs for engineering and maintenance.
Ironically, for a discipline that builds their products with data, we often don’t measure our own performance with data.
How can you assess the maturity of your organization in MLOps? How can you measure how your teams are doing? In this blog, we will propose indicators and metrics to determine the performance of your MLOps practice.
Indicators for MLOps Capability
Implementing MLOps principles is not just a technical exercise. Introducing new tools without having the right skills and practices in place will not improve the team’s performance. Instead, building a strong MLOps capability requires investments across three different areas: people, process, and technology. What are indicators for immature and mature stages across these areas?
Mature MLOps organizations use cross-functional ML teams with well-defined roles, such as data scientists, machine learning engineers, and MLOps engineers. These teams are autonomous and responsible for the solution from inception to production. Regular feedback from end users is essential for success. Short iteration cycles allow for quick improvements. Dedicated MLOps teams help improve efficiency and autonomy by building MLOps platforms and setting out best practices.
How to mature your MLOps Capability: examples of maturity indicators.
These indicators help measure the state of the overall MLOps capability, but how to evaluate the performance of teams across this journey?
Metrics for ML and MLOps Teams
ML teams and MLOps teams have their own focus and their own metrics. Data Scientists and Machine Learning Engineers in ML teams build ML products to solve business problems. MLOps teams enable ML teams by building platforms and setting out best practices.
A central MLOps team supports multiple cross-functional ML teams
The performance of ML teams can be measured with the four software delivery performance metrics defined by DORA (DevOps Research and Assessments). The faster you can make changes to your ML models (‘deployment frequency’ and ‘lead time for changes’ metrics), the sooner you can deliver value to your users and gather their feedback. The more reliable your solutions are (‘change failure rate’ and ‘time to restoration’ metrics), the happier your users will be and the more time your team will have to work on improving models rather than just maintaining them.
MLOps platform teams should focus on ML teams, but their success should not only be measured with the metrics of ML teams. Additional product metrics should show how many teams are using the platform (‘platform adoption’), how satisfied those users are (‘user happiness’), how well it meets their needs (‘capabilities coverage’), and how cost-effective the platform is (‘cost per user’).
Performance metrics for ML and MLOps teams. Adapted from https://dorametrics.org/
For teams building products with data, it is ironic that we often don’t measure our performance with data. These indicators and metrics will make your MLOps practices more data-driven.
Going Beyond MLOps
Interested in assessing your maturity across the full Data & AI scope? Read our whitepaper "AI Maturity Journey"!