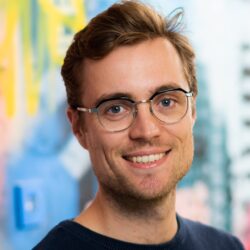
Today, about 80% of companies consider data an essential part of their strategy. However, only 15 out of 100 companies actually succeed in developing AI applications that are adopted and used by the business (according to our 2019/2020 Data & AI Survey).
With businesses heavily invested in data and AI, what makes the difference to be successful with AI? Last year we released a free whitepaper that answers that exact question. Based on our consulting experience and interviews with top Dutch enterprises like Dynniq, ING, Air France-KLM and Randstad we created an AI Maturity Journey framework that helps you grow your organization’s AI Maturity.
In this blog post, we will outline how to use the drivers in the AI Maturity Journey framework to level up and grow your AI competency step by step. The 3 steps are:
- Measure your current practice.
- Do a gap analysis.
- Create an action plan.
Step 1: Measure your current practice
Your organization’s AI Maturity can be measured along two dimensions: your Analytical Capability and your Business Adoption.
The Analytical Capability describes the extent to which your organization is technically able to create AI products. It can be further broken down into three concrete metrics:
- Data: How is data organized and used for building valuable analytics products?
- People & Skills: To what extent does the organization nurture internal talent?
- Tools & Technology: Do the technology stack and tools enable AI innovation?
On the other hand, Business Adoption assesses how well these AI solutions are adopted by and implemented in business processes. The 3 metrics to report on are:
- Executive support: Who is pushing for AI and leading the change?
- Funding: Who is paying for your AI initiatives and the necessary capabilities?
- Implementation: When and how is the business engaged in AI initiatives?
For each of the metrics above, the whitepaper describes what the immature and mature practices are. This provides us with a framework as in Figure 1, where we showed the immature and mature practices for the metric People & Skills in the Analytical Capability domain.
Figure 1: Driver tree showing how AI maturity can be measured by looking into immature and mature practice examples for each of the AI maturity metrics.
Now if you go through each of the practices and score how your organization is doing on a scale from 1 to 5, this will give you insight in the performance of your AI capability.
Consider the following example: an organization that has senior AI professionals in-house, but does not have any vision or strategy on how to stimulate this (and other junior) talent. If, in addition, there is only a very minimal knowledge sharing culture, then we would say that this organization does not know how to retain and nurture their talent. Hence, it would not score very well in the framework.
Figure 2: Example demonstrating how one good practice (with score 5) and two bad practices (with scores of 1 and 2) eventually lead to a negative AI maturity score of 2 for the People & Skills metric.
Doing this exercise for all six metrics will give you a good overall view on where you are in your journey towards becoming an AI driven company.
Step 2: Do a gap analysis
After having scored and assessed your current AI competency, the next step is to set a goal on where you want to grow to. We distinct 4 sequential phases towards being an AI Driven Organization.
- Initialization. Your focus lies on proofing value and discovering what AI can do for your company.
- Continuous Experimentation. You are convinced of the added value of AI and your organization is investing to grow its Analytical Capability.
- Enterprise Empowerment. From your established AI center of excellence you are now decentralizing data science to foster AI opportunities across the organization.
- AI Democratization. In this last step to full maturity you are educating and enabling all your employees to do self-service Analytics and make AI driven decisions.
Figure 3: GoDataDriven’s AI Maturity Journey. The journey towards becoming an AI-Driven Organization is not linear; most organizations first strengthen their Analytical Capability before embedding AI in the business.
As you can see in Figure 3, the journey towards becoming an AI Driven Organization is not a linear one. Your Analytical Capability and Business Adoption are not equally important in each phase, as each phase has a different focus.
This means you cannot jump from the Initialization phase straight to the Enterprise Empowerment phase, without learning the lessons from the Continuous Experimentation phase. Hence, it is essential that you set realistic ambitions with regards to the growth you want to make. Our AI Maturity whitepaper can help you with exactly this.
Based on the outcome of your measurements you should have an idea where you are in the journey. Having set a goal on what you want to achieve in the next 1 to 3 years, you can then use a gap analysis to compare your actual performance with your desired performance.
Step 3: Create an action plan
Understanding which areas you will have to improve on to get to your goal will not bring you there yet; you need to act upon it! In this final step, you need to come up with a roadmap including concrete actions on how to get to your desired AI practice.
Figure 4 shows such an example of a roadmap that we created for one of our clients based on an AI Maturity assessment. The audit revealed that there was work to do on both the strategic (Business Adoption) side as well as the technical (Analytical Capability) side.
The ultimate vehicle to tackle many of the problems was to set up Data Science and Data Engineering communities of practice, wherein AI professionals could work together to improve their ways of working. In parallel, we worked on the organization’s AI strategy and AI Solution Framework to get a better alignment between Business and AI teams.
Figure 4: Example of a roadmap outlining concrete actions along a strategical and technical track in order to improve AI Maturity.
Make the AI Maturity Journey work for you
In this blog post, we outlined a 3 step process towards becoming an AI-driven organization. This approach becomes extremely effective if you repeat it every year to evaluate whether you made progression and to determine your next steps.
We know it is not easy to objectively measure and criticize your own practice. If you need assistance, our AI Maturity Scan could be an option for you. Within a period of only three weeks, we will do many interviews as well as code and architecture deep dives. We have an extensive framework with metrics to score your practice on, giving you detailed insight in how you are doing. Included in the final report are the advised next steps on how to proceed.
If you want to read more about how to make the AI Maturity Journey work for you.
I hope the blog post will help you become more successful with Data & AI!
Please don’t hesitate to contact us in case of questions.